Demo: Velodyne dataset in Rawlog format, 3D-LiDAR SLAM
This demo shows how to launch a 3D LiDAR SLAM system reading Velodyne scans from an MRPT .rawlog file.
To run this demo, download the example dataset: sample_rawlog1 (part of sample_dataset).
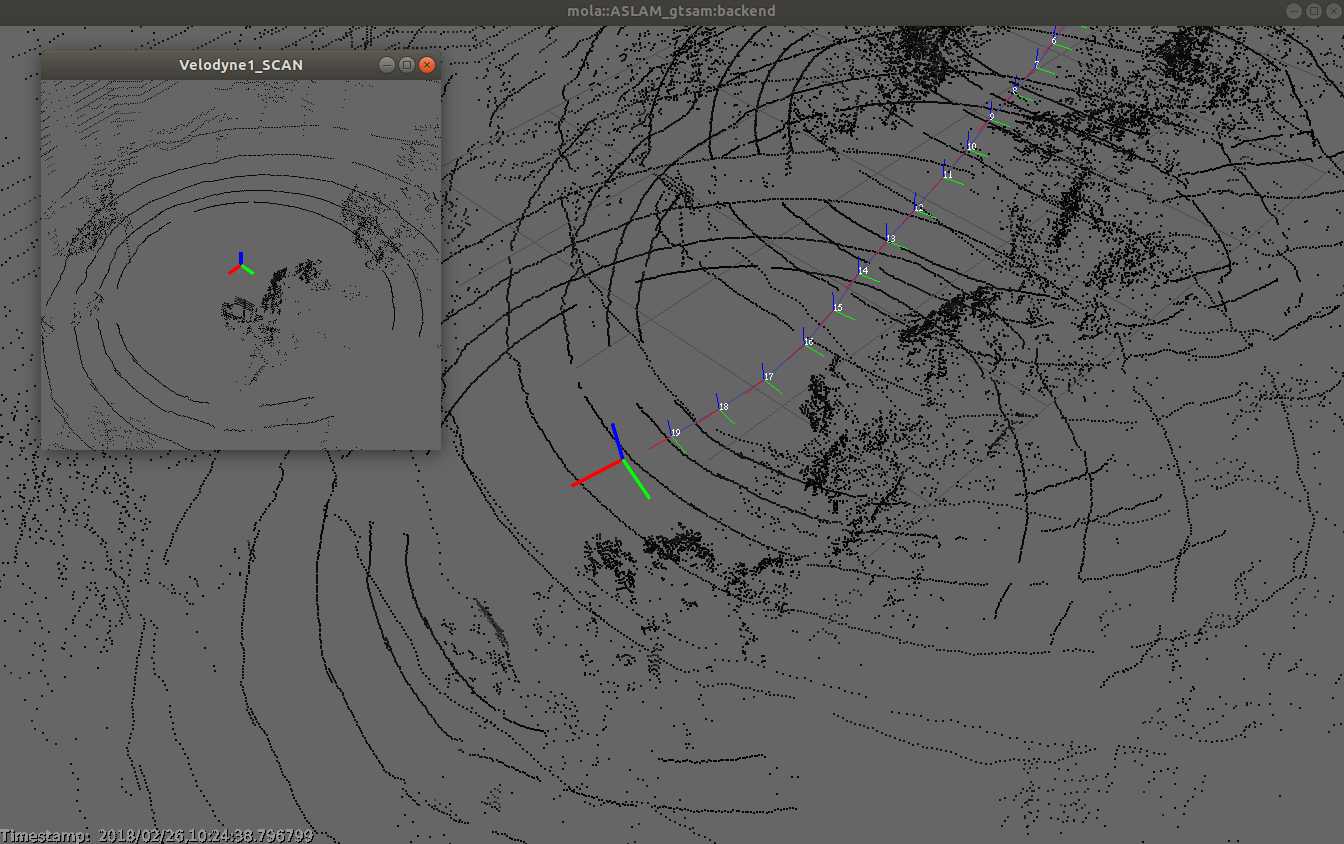
Usage
cd mola/demos
MOLA_INPUT_RAWLOG=map1_test1.rawlog mola-cli rawlog_odom_and_lidar.yml -p